A Comprehensive Guide to a Career as a Big Data Engineer
In this guide, learn everything you need to know about a career as a Big Data Engineer.
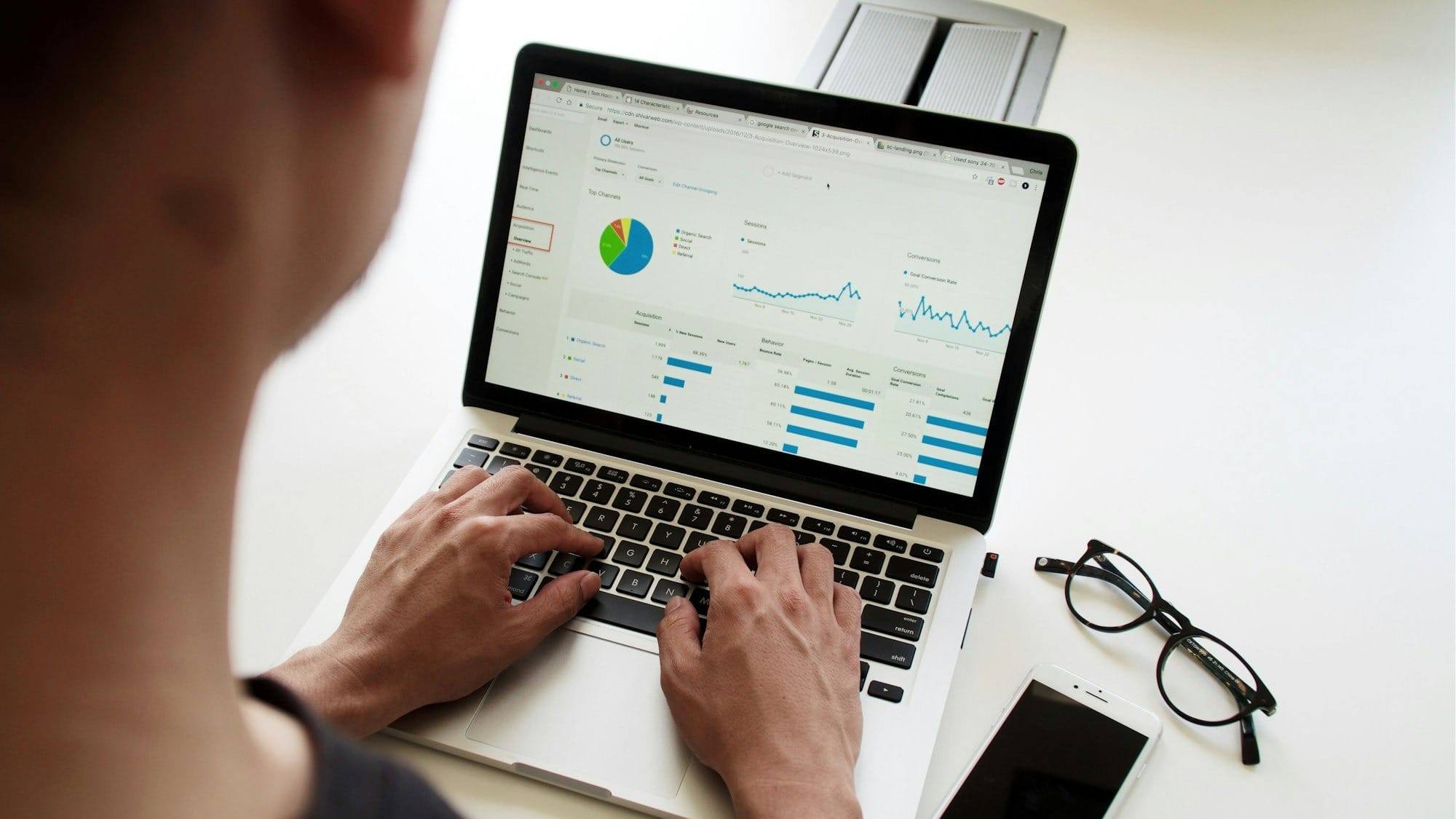
In today’s data-driven world, the ability to effectively manage and process large amounts of data is critical for organizations across all industries. As businesses increasingly rely on data for decision-making, the need for specialized roles in data management has grown. One such role is that of a Big Data Engineer.
Big Data Engineers play a crucial part in enabling companies to harness the power of data for analytical purposes, often working with complex tools and frameworks to build scalable and efficient data systems.
Who is a Big Data Engineer?
A Big Data Engineer is an expert responsible for designing, building, and maintaining the architecture that allows large sets of data to be processed and analyzed. They work with tools like Hadoop, Apache Spark, Kafka, and other big data technologies to create systems that can store and process vast amounts of information. Their work supports data scientists and analysts by preparing data for analysis, ensuring data availability, and ensuring the systems can scale as data grows.
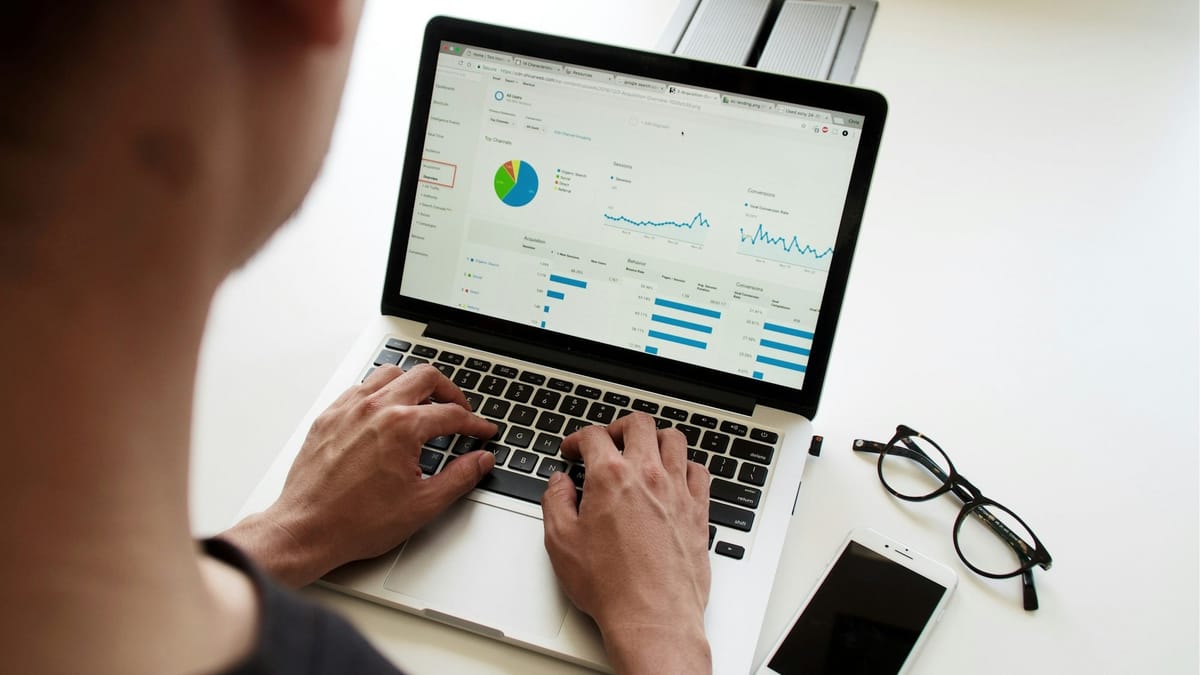
How Much Does a Big Data Engineer Earn?
The salary of a Big Data Engineer can vary depending on factors like experience, location, industry, and company. According to Glassdoor, a Big Data Engineer can earn between $105,000 and $156,000, but on average, Big Data Engineers earn $127,000 annually.
Role of a Big Data Engineer?
The role of a Big Data Engineer typically involves several key responsibilities:
- Data Pipeline Development: Building and optimizing data pipelines that handle large-scale data processing.
- Data Integration: Integrating data from various sources such as databases, APIs, and real-time data streams into centralized storage systems.
- Data Storage Management: Managing distributed data storage systems like Hadoop HDFS, Amazon S3, or cloud-based storage.
- Data Processing: Using tools like Apache Spark, Hadoop, and Kafka to process data in real-time or batch modes.
- Data Quality and Governance: Ensuring that data is clean, accurate, and complies with governance and privacy regulations.
- Collaboration: Working closely with data scientists, analysts, and business teams to understand data needs and provide them with usable, high-quality data.