Business Analysis vs. Data Analysis: Understanding the Key Differences
In this article, compare and contrast these two disciplines.
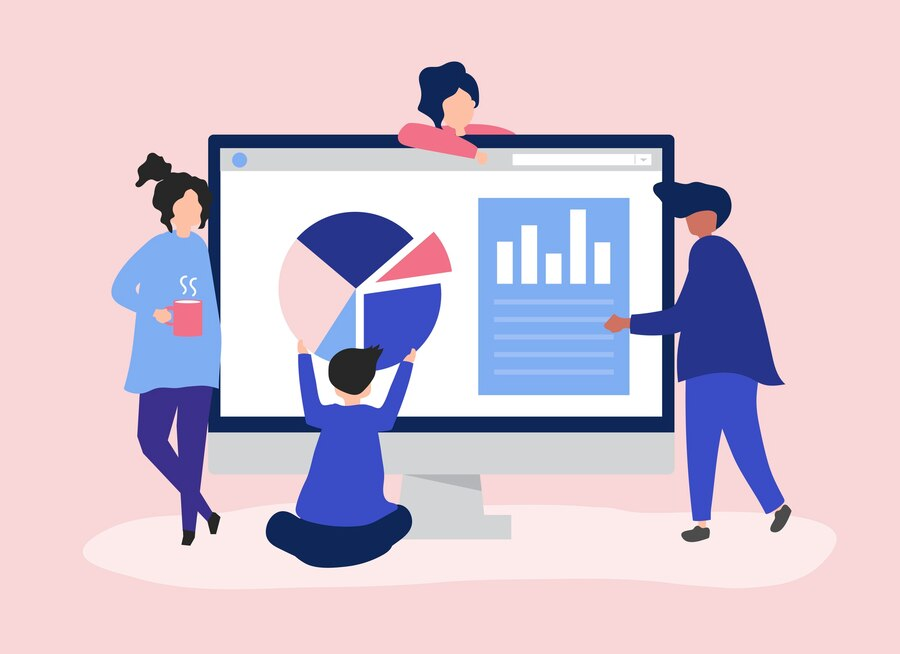
In today's data-driven business environment, business analysis, as well as data analysis, is important in helping organizations understand information, identify opportunities and make better decisions.
Both of these fields differ, nonetheless, in their emphasis, objectives, and methods of approach to reach them. Companies looking to use business and data analytics capabilities more effectively need to understand these differences.
In this article, we will take a look at the main definitions, goals, and activities of the business analysis and data analysis roles. This will compare and contrast these two disciplines in order to show their individual value propositions. It will also discuss trends in each field and recommend how to synergistically combine business analysis services and data analysis skillsets to generate more business impact.
Defining Business Analysis
Business analysis is the practice of analyzing an organization’s business needs and problems to help come up with solutions and drive change. A business analyst usually acts as a bridge between business people and technical teams.
The core objectives of business analysis include:
- Clarifying business requirements, needs, and pain points
- Assessing the business impact of issues and opportunities
- Identifying potential solutions and options
- Evaluating solution alternatives and trade-offs
- Creating business cases to justify projects and expenditures
- Managing solution implementation plans and business change enablement
To accomplish these goals, some common business analysis activities include:
- Interviewing the business stakeholders and working with them in the form of workshops, etc.
- Analyzing businesses of interest in terms of business context, industry trends, etc.
Bbusiness analysis methods of modeling business processes, requirements, gaps and business analytics future states.
- Qualitative business techniques such as SWOT analysis and root cause analysis are performed.
- Business analyst methodologies such as cost-benefit analysis, total cost of ownership models.
- Filing findings in artifacts like the business requirements document and the solution recommendations document.
As we’ll explore next, while some business analysis techniques and skills relate to data analysis, business analysts usually focus more holistically across process, organizational, cultural, and ecosystem perspectives rather than just quantitative data.
Defining Data Analysis
Basically, Data analysis is the quantitative statistical study of datasets, metrics, and information to find insights, patterns and recommendations. A data analyst uses a wide range of mathematical and analytical techniques in business to transform raw data into meaningful interpretations that serve as a guide for business strategy and objectives.
Some of the main goals of data analysis include:
- Integrating and cleaning large volumes of data
- Manipulating data using statistical programming languages
- Using machine learning algorithms or predictive modeling business analysis tools and techniques
- Identifying trends, correlations, behaviors, and insights in the data
- Data visualization using charts, graphs, and dashboards
- Concluding and identifying data-driven opportunities
- Communicating data findings to business leaders to drive decisions
Common analysis data activities thus center on leveraging advanced analytics skillsets to make the most of data resources. These include:
- Blending and cleaning large datasets and accessing them.
- A collection of coding scripts for statistical analysis in Python, R, SQL, etc.
- Machine learning algorithm selection and application for the purpose of pattern recognition and predictive modeling.
- Data such as performing regression analysis, significance testing, clustering, text mining etc.
- Making interactive Tableau or Qlik Sense dashboards for data visualization.
- Converting data analysis results in interpretations and main points being taken away.
Next, we’ll see that while data analysts spend hours honing their advanced quantitative skills, their perspective may be more restricted than that of the business analyst, who takes into account a variety of business concepts, processes, and qualitative considerations.
Key Differences and Distinctions
Business analysis and data analysis overlap in the areas of skill sets but are also quite different in terms of focus areas, objectives and methods. Key distinctions include:
Orientation: Business vs. Data
On the other hand, business analysis is business oriented and data analysis is data and technical oriented. Business analysts study the key aspects of the business: its core functions, processes, organizational and dynamic structure, pain points and objectives. Data analysts immerse themselves in the data – accessing, preparing, manipulating, modeling, interpreting and visualizing data.
Breadth vs. Depth of Focus
Second, business analysts tend to have a broader focus across various facets of the business, while data analysts drill down deeper into the data. Business analysts need to understand interactions between business processes, organizational groups, technologies, metrics, and ecosystems. This “big picture” view allows them to facilitate cross-functional solutions. Data analysts narrow their perspective to maximize insight from the volumes of data – they finely comb through the data to tease trends, correlations, and insights.
Types of Analysis: Qualitative vs. Quantitative
A second key difference is the type of analysis performed. Business analyst tools and techniques are mostly good at qualitative solutions, such as understanding business needs, mapping processes, analyzing gaps, identifying improvement opportunities, recommending solutions and analyzing the impact of changes. Quantitative analysis is the work of data analysts, who perform mathematical and statistical techniques to glean insights from behavior, patterns, and relationships in data to make predictions.
Scope: Strategic vs. Operational
Additionally, the scope differs for business and data analysis. Business analysts usually operate at a more strategic level, clarifying business goals, initiatives, and capabilities needed for the future of business analytics state. Data analysts work at a more tactical level, focusing on tangible metrics, KPIs, and information that can guide day-to-day and short-term decisions and operations.
Deliverables: Requirements vs. Analytics
The outputs also differ. Requirement documents, process diagrams, cost-benefit comparisons, roadmaps, and recommendations to guide business change are produced by business analysts. Data analysts produce cleaned datasets, statistical analysis results, predictive models, and interactive Power BI dashboards to give decision-makers data-driven intelligence.
Skillsets: Business vs. Technical
Finally, there is little overlap in core skills. Business analysis methodologies require strategic thinking, contextual understanding, problem-solving, workshop facilitation, process modeling, qualitative assessment, and project planning skills. Data analytics centers on statistical programming, data manipulation, algorithm expertise, information modeling, dashboard design, advanced mathematics, and coding capabilities. their
While focuses differ, business analysis paired with data analysis can be an incredibly powerful combination for driving optimal business decisions and outcomes, as the next section will discuss.
Trends and Developments
Both business analysis and data analytics fields continue to evolve rapidly to deliver more value in the modern, quick-paced digital economy. Some key trends include:
More Demand for Business and Data Insights
Overall, organizations are aware of the essential role of business insights and data-driven decision-making. A 2023 Gartner survey discovered that the heads of data and analytics report to the CEO in 44 percent of organizations, up from 13 percent in 2017, pointing to the strategic clout of data-driven insights. Most companies plan to invest further in business analysis and data analytics capabilities.
Emergence of “Hybrid” Skillsets
Rather than stark divisions, overlaps between business analytics techniques and data analysis continue to grow. Many analyst positions now look for “hybrid” skill sets - business analysts with data analytics capabilities and data scientists with business analysis chops. Domain knowledge plus analytical chops promise to uncover more impactful insights.
More Focus on Enabling Business Change
Business analysts are increasingly prioritizing capabilities beyond just gathering requirements and assessing processes. There is a greater emphasis on project planning, communications, and business readiness to drive the adoption of solutions. Data analysts also now need to interpret and clearly communicate findings rather than just deliver data outputs.
Expansion Across More Business Functions
Business analyst techniques, once more concentrated in IT departments, are now being leveraged across functions like operations, finance, marketing, HR, and strategy to assess needs and drive transformation initiatives. Data analytics also continues to expand from just marketing into wider applications.
Continued Growth of Data Analytics
Organizations are looking to become insight-driven, and data analytics talent is still in high demand. As analytics and data management jobs continue to grow, Gartner predicts that by 2028, 75 percent of enterprises will have moved from piloting to fully operationalizing AI. The power of current trends in business analytics is to connect insights to what’s important for business.
Recommendations for Maximizing Value
Business analysis and data analysis are fundamentally different, but when you combine them thoughtfully, you can make better business decisions and results. Here are the best practices to consider:
Foster Collaboration Between Groups
Facilitate collaboration through meetings, working sessions, and project work rather than keeping business analysts and data scientists siloed. Collaboration allows them to align their analysis with business objectives better.
Promote Visibility Into Capabilities
It helps business analysts and data analysts to understand each other’s capabilities, approaches and limitations. By doing this, task allocation will be appropriate, and outputs will be set accordingly. This cross-training between groups can create mutual understanding.
Define Problems and Questions Collaboratively
Both sets of analysts should be involved in framing the problem context and defining the key question the analysis needs to address. This will also help us to focus the effort on meaningful rather than speculative insights.
Map Analytical Insights to Business Impact
Data analysts are required to articulate insights in business terms like performance gains, cost savings, and revenue upside rather than just data differences. This drives adoption and action based on analysis.
Enrich Analysis Through Outside-In Perspectives
While data provides historical internal intelligence, it incorporates outside ecosystems, customer preferences, industry benchmarks, and market trends to enrich analysis and recommendations.
Connect Analysis to Business Change Roadmaps
Tie analytical insights to clear implementation plans outlining how people, processes, and systems will change. Who needs to act on recommendations? What will adoption require? The business analysis brings critical change enablement.
Evolve Analysis Into Continuous Intelligence Cycles
Rather than one-off analyses, embed feedback loops in which business metrics and measures inform ongoing analyses to course-correct strategy and operations.
Conclusion
So, what is the future of business analytics and data analytics in your organization? Understanding the different dimensions of an organization to uncover opportunities requires business and data analysis working together.
Although the focus of their core is different, combining data-driven intelligence with the business context and qualitative factors will give more comprehensive insights and enable significant performance gains.
Business and data analytics experts are a unique value add for companies, and companies need to leverage the unique value add of business and data analytics while helping business and data analytics experts collaborate with each other to achieve shared objectives.
Business analysis combined with data science has the potential to take organizations to new heights of prosperity and competitiveness when there is the right synergy between the two.